The workshop on Statistics in Metric Spaces was held at ENSAE, on October 11, 12 and 13, 2023. It brought together international experts in the joint fields of statistics, optimization, probability theory and geometry. Each participant gave a 45-60 min talk and the range of topics that were covered was broad, tackling modern questions concerning statistical analysis on non-standard spaces.
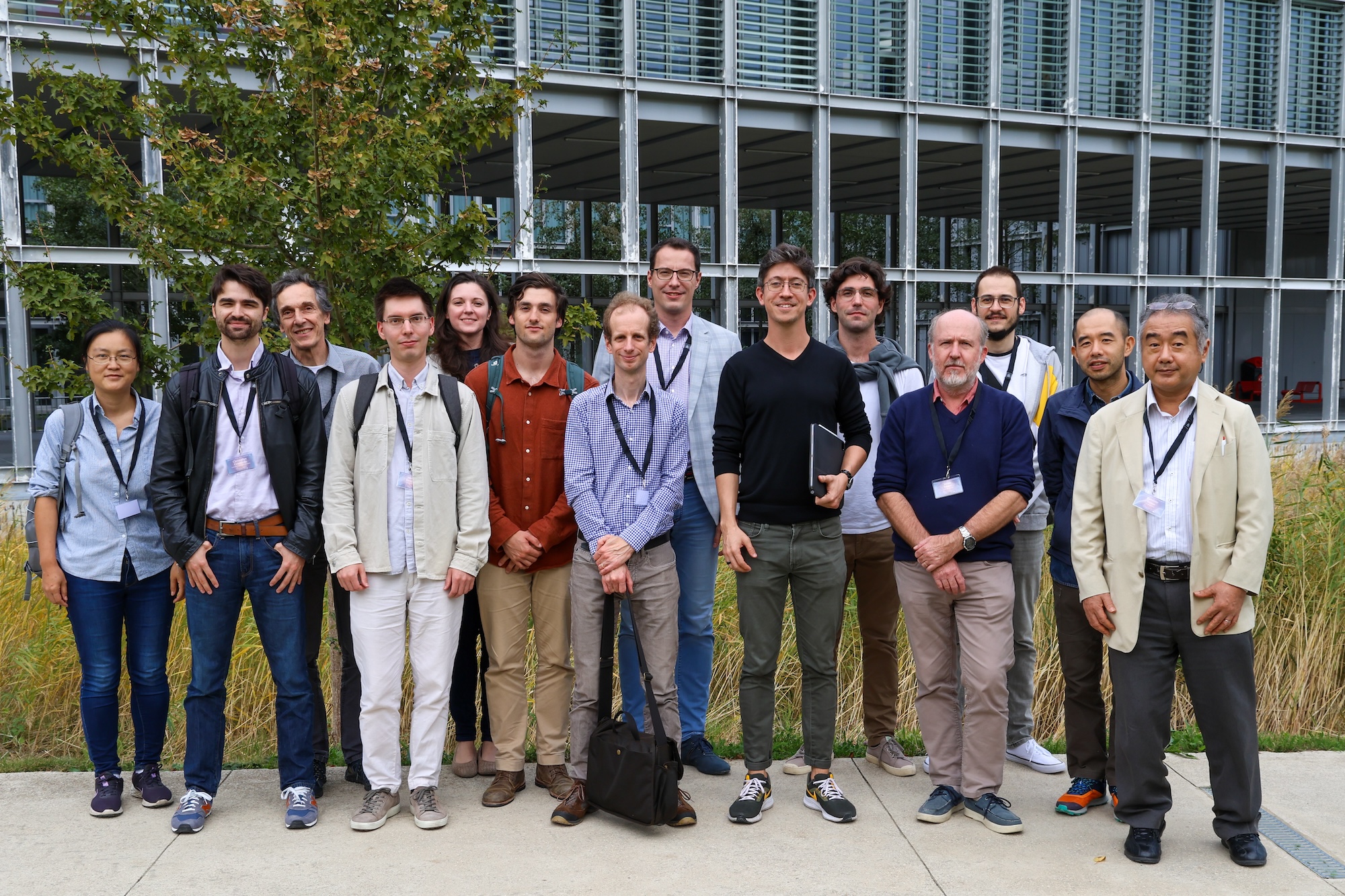
Victor-Emmanuel Brunel (CREST-ENSAE), Christopher Criscitiello (EPFL), Stephan Huckemann (Georg-August-Universität Göttingen), Alexey Kroshnin (Weierstrass-Institut Für Angewandte Analysis und Stochastik), Kazuhiro Kuwae (Fukuoka University), Tom Nye (Newcastle University), Shin-ichi Ohta (Osaka University), Miklós Pálfia (Corvinus University of Budapest), Pierre Pansu (Université Paris-Saclay), Quentin Paris (HSE University), Xavier Pennec (INRIA), Gabriel Romon (CREST-ENSAE), Jordan Serres (CREST-ENSAE), Austin Stromme (CREST-ENSAE)
While available data become more and more rich and complex, it is essential to understand their intrinsic geometry, for instance as a tool of dimensionality reduction or, sometimes, in order to produce interpretable statistical procedures. This, however, also comes at a cost, since these geometries may be non-standard (e.g., non-linear and/or non-smooth geometries), yielding new challenges from the points of view of both statistical and algorithmic analysis.
For instance, directional data lie on spheres or projective spaces. In shape statistics, data are encoded as landmarks on three-dimensional objects, which should be invariant under rigid transformations: Hence, data lie in the quotient of a Euclidean space by a class of rigid transformations. In fact, such quotient spaces are also useful to understand statistical models that arise in econometrics, when a parameter is only identifiable up to some known transformations. Optimal transport theory is based on Wasserstein spaces, which are metric spaces with Riemannian/Finsler-like geometries. In various fields, in particular physics and economics, the geometry provided by optimal transport on sets of probability measures has been shown to be very well adapted to understand general phenomena, such as transportation of goods, or distribution of tasks, capital, etc. In the machine learning community, it has also been recently pointed out that metric trees and hyperbolic spaces, which exhibit negative curvature, are well adapted to encode data with hierarchical structures.
While probability theory is now fairly well understood in smooth, finite dimensional spaces (such as Euclidean spaces and Riemannian manifolds), much less is known in more general metric spaces, exhibiting possible infinite dimension (such as functional spaces), inhomogeneous structure (such as stratified spaces), etc. From a more algorithmic prospective, gradient flows and their discretization in non-smooth spaces are challenging because they require brand new approaches (e.g., new definitions of (sub)-gradients), yet they are essential in order to extend fundamental tools such as gradient descent algorithms to non-standard setups. Even in smooth spaces, the impact of curvature on gradient descent algorithms is still not clearly understood. More generally, the notion of convexity, which is pervasive to probability theory, statistical learning and optimization, and its interplays with curvature, still raise challenging questions.
To summarize, the impact of curvature (or generalized notions of curvature) on measure concentration, on the statistical behavior of learning algorithms and on their computational aspects is a flourishing topic of research that brings together experts in smooth/non-smooth geometry, statistics, probability theory and optimization.
The workshop brought these challenges onto the stage, and yielded fruitful discussions among the participants and the audience, with the goal of entailing future collaborations. We hope that this workshop, on Statistics in Metric Spaces, was the first edition of a long series, that will also spread interest in these rich topics into a broader audience.