Une interview de Vianney Perchet, coordinateur du projet BOLD, qui vise à améliorer l’apprentissage séquentiel
18.04.2024
Arnak Dalalyan and Vianney Perchet nominated as new members of the editorial board of the journal “Foundations and Trends® in Machine Learning”.
Arnak Dalalyan and Vianney Perchet nominated as new members of the editorial board of the journal “Foundations and Trends® in Machine Learning”.
Arnak Dalalyan and Vianney Perchet nominated as new members of the editorial board of the journal “Foundations and Trends® in Machine Learning”.
Arnak Dalalyan and Vianney Perchet nominated as new members of the editorial board of the journal “Foundations and Trends® in Machine Learning”.
Catalyzing Conversation: The Royal Statistical Society’s Webinar on Dalalyan’s Paper ‘Theoretical Guarantees for Approximate Sampling from Smooth and Log-Concave Densities'”
On 31 October, the Royal Statistical Society webinar was devoted to Arnak S. Dalalyan’s 2017 Series B paper ‘Theoretical Guarantees for Approximate Sampling from Smooth and Log-Concave Densities’, featuring contributions from Hani Doss and Alain Durmus.
“[Dalalyan] combines techniques from convex optimisation with insights from random processes to provide non-asymptotic guarantees regarding the accuracy of sampling from a target probability density. These guarantees are notably simpler than those found in the existing literature, and they remain unaffected by dimensionality.
The findings pave the way for more widespread adoption of the mathematical and algorithmic tools developed in the field of convex optimization within the domains of statistics and machine learning.”
Showcasing significant recent papers published in the Society’s journals, the journal webinar format aims to bring authors closer to their audience in academia and industry. Impactful features of the paper are presented by the author, followed by contributions from the guest discussants.
Catalyzing Conversation: The Royal Statistical Society’s Webinar on Dalalyan’s Paper ‘Theoretical Guarantees for Approximate Sampling from Smooth and Log-Concave Densities'”
On 31 October, the Royal Statistical Society webinar was devoted to Arnak S. Dalalyan’s 2017 Series B paper ‘Theoretical Guarantees for Approximate Sampling from Smooth and Log-Concave Densities’, featuring contributions from Hani Doss and Alain Durmus.
“[Dalalyan] combines techniques from convex optimisation with insights from random processes to provide non-asymptotic guarantees regarding the accuracy of sampling from a target probability density. These guarantees are notably simpler than those found in the existing literature, and they remain unaffected by dimensionality.
The findings pave the way for more widespread adoption of the mathematical and algorithmic tools developed in the field of convex optimization within the domains of statistics and machine learning.”
Showcasing significant recent papers published in the Society’s journals, the journal webinar format aims to bring authors closer to their audience in academia and industry. Impactful features of the paper are presented by the author, followed by contributions from the guest discussants.
CRESTive Minds – Épisode 3 – Anna Korba
Researcher portrait: Anna Korba, assistant professor at CREST-ENSAE Paris.
What is your career path?
I pursued a three-year program in Math/Data Science at ENSAE, concurrently completing a specialized Master’s in Machine Learning at ENS Cachan. My academic journey continued with a Ph.D. in Machine Learning at Télécom ParisTech under the supervision of Stephan Clémençon.
Afterward, I gained valuable experience as a postdoctoral researcher at the Gatsby Computational Neuroscience Unit, University College London, collaborating with Arthur Gretton.
In 2020, I returned to ENSAE, joining the Statistics Department as an Assistant Professor. This trajectory has equipped me with a strong foundation in both Machine Learning and Statistics.
Did you have a statistician who particularly inspired you? If so, what were their research topics?
While I don’t have a single statistician who profoundly influenced me, I draw inspiration from the excellent mathematics taught by instructors like Arnak Dalalyan, Nicolas Chopin, Cristina Butucea and others at ENSAE. Also, I remember very well my first international conference in Machine Learning (ICML 2015 in Lille). Attending talks within the Deep Learning community, though somewhat distant from my research focus at the time, left a lasting impression. Witnessing the rapid and substantial advancements, particularly in areas like question answering, fascinated me. Conferences I attended provided exposure to influential figures—from esteemed senior professors to brilliant Ph.D. students—enriching my perspective on various statistics and machine learning subjects.
How did you get into statistics and Machine Learning in particular?
As a student I liked mathematics and coding. At ENSAE, I had the choice between quantitative finance and machine learning. With quantitative finance hiring slowing down, I embraced the rising tide of machine learning, drawn to its dynamic nature and innovative potential.
What are your research topics?
One of my primary research focuses is on sampling—approximating a target probability distribution when only partial information is available, such as its unnormalized density or samples. This versatile problem holds applications in various areas of machine learning.
In Bayesian inference, I address the posterior probability distribution over model parameters, particularly in supervised learning scenarios like determining the weights of linear or neural network regressors. Additionally, in generative modeling, my work involves learning the underlying process from a set of samples, such as true faces from celebrities, with the goal of generating new faces.
Beyond sampling, I’ve contributed to research in preference learning, structured prediction, and causality.
The framework of your field of research is fairly recent, and brings together different communities. Could you name them and explain how this collaborative effervescence has enabled a great advance?
My research intersects various communities, including experts in MCMC (Markov Chain Monte Carlo) methods, partial differential equations, dynamical systems, optimal transport (OT), and machine learning. In recent years, these traditionally independent fields have converged, fostering collaborative efforts.
A significant milestone in this convergence was a semester at Berkeley, organized by P. Rigollet, S. Di Marino, K. Craig, and A. Wilson, which brought together researchers from these diverse areas. Since then, the boundaries between these communities have become more fluid, sparking heightened interest and collaboration.
For example, I co-presented a tutorial on Wasserstein gradient flows with Adil Salim at ICML 2022, while Marco Cuturi and Charlotte Bunne presented a tutorial on OT, control, and dynamical systems at ICML 2023. These tutorials aim to introduce promising research directions and tools, providing a comprehensive panorama to a broad audience of machine learning researchers.
This collaborative effervescence has resulted in exciting progress on both theoretical and computational fronts. Researchers with expertise in multiple domains are leveraging their backgrounds to overcome challenges, offering convergence guarantees for numerical schemes and addressing practical limitations in sampling schemes, such as convergence time and local minima.
There are still many unsolved problems in the various applications. What would you like to solve or advance in your future research?
While significant strides have been made in sampling techniques inspired by optimization literature, there are still numerous unexplored aspects. My current research focus involves the incorporation of constraints into sampling methodologies. For instance, I am exploring ways to ensure fairness in predictive models by constraining the posterior distribution, making predictions independent of sensitive attributes like gender. In the realm of generative modeling, it is interesting to incorporate constraints or rewards as well, e.g. to generate images that satisfy some criterion such as brightness.
How is the intersection of fair analysis methods and Bayesian statistical methods an important advance for Machine Learning?
Bayesian inference, by providing a posterior distribution over the parameters of a model, allows for predictions with uncertainty. This is pivotal in applications where users require models capable of predicting with uncertainty, as the distribution over predictions provides a more comprehensive understanding than pointwise predictions alone. Moreover, incorporating fairness constraints in Bayesian methods holds important applications, ensuring that predictions are not influenced by sensitive attributes. This intersection enhances the interpretability and ethical considerations of machine learning models.
CRESTive Minds – Épisode 3 – Anna Korba
Researcher portrait: Anna Korba, assistant professor at CREST-ENSAE Paris.
What is your career path?
I pursued a three-year program in Math/Data Science at ENSAE, concurrently completing a specialized Master’s in Machine Learning at ENS Cachan. My academic journey continued with a Ph.D. in Machine Learning at Télécom ParisTech under the supervision of Stephan Clémençon.
Afterward, I gained valuable experience as a postdoctoral researcher at the Gatsby Computational Neuroscience Unit, University College London, collaborating with Arthur Gretton.
In 2020, I returned to ENSAE, joining the Statistics Department as an Assistant Professor. This trajectory has equipped me with a strong foundation in both Machine Learning and Statistics.
Did you have a statistician who particularly inspired you? If so, what were their research topics?
While I don’t have a single statistician who profoundly influenced me, I draw inspiration from the excellent mathematics taught by instructors like Arnak Dalalyan, Nicolas Chopin, Cristina Butucea and others at ENSAE. Also, I remember very well my first international conference in Machine Learning (ICML 2015 in Lille). Attending talks within the Deep Learning community, though somewhat distant from my research focus at the time, left a lasting impression. Witnessing the rapid and substantial advancements, particularly in areas like question answering, fascinated me. Conferences I attended provided exposure to influential figures—from esteemed senior professors to brilliant Ph.D. students—enriching my perspective on various statistics and machine learning subjects.
How did you get into statistics and Machine Learning in particular?
As a student I liked mathematics and coding. At ENSAE, I had the choice between quantitative finance and machine learning. With quantitative finance hiring slowing down, I embraced the rising tide of machine learning, drawn to its dynamic nature and innovative potential.
What are your research topics?
One of my primary research focuses is on sampling—approximating a target probability distribution when only partial information is available, such as its unnormalized density or samples. This versatile problem holds applications in various areas of machine learning.
In Bayesian inference, I address the posterior probability distribution over model parameters, particularly in supervised learning scenarios like determining the weights of linear or neural network regressors. Additionally, in generative modeling, my work involves learning the underlying process from a set of samples, such as true faces from celebrities, with the goal of generating new faces.
Beyond sampling, I’ve contributed to research in preference learning, structured prediction, and causality.
The framework of your field of research is fairly recent, and brings together different communities. Could you name them and explain how this collaborative effervescence has enabled a great advance?
My research intersects various communities, including experts in MCMC (Markov Chain Monte Carlo) methods, partial differential equations, dynamical systems, optimal transport (OT), and machine learning. In recent years, these traditionally independent fields have converged, fostering collaborative efforts.
A significant milestone in this convergence was a semester at Berkeley, organized by P. Rigollet, S. Di Marino, K. Craig, and A. Wilson, which brought together researchers from these diverse areas. Since then, the boundaries between these communities have become more fluid, sparking heightened interest and collaboration.
For example, I co-presented a tutorial on Wasserstein gradient flows with Adil Salim at ICML 2022, while Marco Cuturi and Charlotte Bunne presented a tutorial on OT, control, and dynamical systems at ICML 2023. These tutorials aim to introduce promising research directions and tools, providing a comprehensive panorama to a broad audience of machine learning researchers.
This collaborative effervescence has resulted in exciting progress on both theoretical and computational fronts. Researchers with expertise in multiple domains are leveraging their backgrounds to overcome challenges, offering convergence guarantees for numerical schemes and addressing practical limitations in sampling schemes, such as convergence time and local minima.
There are still many unsolved problems in the various applications. What would you like to solve or advance in your future research?
While significant strides have been made in sampling techniques inspired by optimization literature, there are still numerous unexplored aspects. My current research focus involves the incorporation of constraints into sampling methodologies. For instance, I am exploring ways to ensure fairness in predictive models by constraining the posterior distribution, making predictions independent of sensitive attributes like gender. In the realm of generative modeling, it is interesting to incorporate constraints or rewards as well, e.g. to generate images that satisfy some criterion such as brightness.
How is the intersection of fair analysis methods and Bayesian statistical methods an important advance for Machine Learning?
Bayesian inference, by providing a posterior distribution over the parameters of a model, allows for predictions with uncertainty. This is pivotal in applications where users require models capable of predicting with uncertainty, as the distribution over predictions provides a more comprehensive understanding than pointwise predictions alone. Moreover, incorporating fairness constraints in Bayesian methods holds important applications, ensuring that predictions are not influenced by sensitive attributes. This intersection enhances the interpretability and ethical considerations of machine learning models.
Advances in Bayesian Computation: A Masterclass on State-Space Models and Sequential Monte Carlo Algorithms by Professor Nicolas Chopin
Nicolas Chopin, Professor in Data Sciences / Statistics / Machine Learning
Nicolas Chopin is a Professor of Data Sciences/Statistics/Machine Learning at ENSAE Paris, Institut Polytechnique de Paris, and researcher at CREST.
He is particularly interested in all aspects of Bayesian computation, that is algorithms to perform Bayesian inference, including:
- Monte Carlo methods: particularly Sequential Monte Carlo, but also plain, quasi- and Markov chain Monte Carlo;
- Fast approximations: e.g. Expectation Propagation and variational Bayes.
Nicolas Chopin is currently an Associate editor of two journals, Annals of Statistics and Biometrika.
Last October, Nicolas Chopin was invited to give 2 Master Classes during the Autumn School in Bayesian Statistics.
Autumn School in Bayesian Statistics 2023
The objective of this autumn school is to provide a comprehensive overview of Bayesian methods for complex settings: modeling techniques, computational advances, theoretical guarantees, and practical implementation. It will include two masterclasses, on Sequential Monte Carlo and on Bayesian causal inference, tutorials on NIMBLE and on Bayesian Statistics with Python, and a selection of invited and contributed talks.
More information on the Autumn School in Bayesian Statistics 2023
Masterclass given by Nicolas Chopin on State-Space Models and SMC Algorithms
Nicolas Chopin gave a four-hour master class on state-space models and SMC algorithms (Sequential Monte Carlo, also known as particle filters) as part of the “Bayes at CIRM” autumn school, held at CIRM (CNRS permanent conference center for mathematics) from October 30 to November 3, 2023. State-space models have become very popular in recent years in all fields of application where a dynamic system is imperfectly observed, including epidemiology (modeling an epidemic such as COVID), robotics (navigation), finance (stochastic volatility), automatic language processing (grammatical function detection), and even image generation (diffusion-based methods). These models are particularly difficult to estimate. SMC algorithms have been developed over the last twenty years to meet this challenge. More recently, they have been extended to a more general class of problems and can now also be used to simulate any probability law, as effectively or even more effectively than MCMC (Markov chain Monte Carlo) algorithms.
In four hours, the master class presents an overview of all aspects of research in this field, from theory (convergence of algorithms), through the development of new algorithms and their efficient implementation in Python, to their application in different fields.
This master class is based on the speaker’s book (co-authored with Omiros Papaspiliopoulos): “An introduction to Sequential Monte Carlo” published by Springer :
https://link.springer.com/book/10.1007/978-3-030-47845-2
The master class was video-recorded and uploaded by CIRM on YouTube:
https://www.youtube.com/watch?v=0CpY1WdTkFE
https://www.youtube.com/watch?v=uuoGTBy1yH8
Advances in Bayesian Computation: A Masterclass on State-Space Models and Sequential Monte Carlo Algorithms by Professor Nicolas Chopin
Nicolas Chopin, Professor in Data Sciences / Statistics / Machine Learning
Nicolas Chopin is a Professor of Data Sciences/Statistics/Machine Learning at ENSAE Paris, Institut Polytechnique de Paris, and researcher at CREST.
He is particularly interested in all aspects of Bayesian computation, that is algorithms to perform Bayesian inference, including:
- Monte Carlo methods: particularly Sequential Monte Carlo, but also plain, quasi- and Markov chain Monte Carlo;
- Fast approximations: e.g. Expectation Propagation and variational Bayes.
Nicolas Chopin is currently an Associate editor of two journals, Annals of Statistics and Biometrika.
Last October, Nicolas Chopin was invited to give 2 Master Classes during the Autumn School in Bayesian Statistics.
Autumn School in Bayesian Statistics 2023
The objective of this autumn school is to provide a comprehensive overview of Bayesian methods for complex settings: modeling techniques, computational advances, theoretical guarantees, and practical implementation. It will include two masterclasses, on Sequential Monte Carlo and on Bayesian causal inference, tutorials on NIMBLE and on Bayesian Statistics with Python, and a selection of invited and contributed talks.
More information on the Autumn School in Bayesian Statistics 2023
Masterclass given by Nicolas Chopin on State-Space Models and SMC Algorithms
Nicolas Chopin gave a four-hour master class on state-space models and SMC algorithms (Sequential Monte Carlo, also known as particle filters) as part of the “Bayes at CIRM” autumn school, held at CIRM (CNRS permanent conference center for mathematics) from October 30 to November 3, 2023. State-space models have become very popular in recent years in all fields of application where a dynamic system is imperfectly observed, including epidemiology (modeling an epidemic such as COVID), robotics (navigation), finance (stochastic volatility), automatic language processing (grammatical function detection), and even image generation (diffusion-based methods). These models are particularly difficult to estimate. SMC algorithms have been developed over the last twenty years to meet this challenge. More recently, they have been extended to a more general class of problems and can now also be used to simulate any probability law, as effectively or even more effectively than MCMC (Markov chain Monte Carlo) algorithms.
In four hours, the master class presents an overview of all aspects of research in this field, from theory (convergence of algorithms), through the development of new algorithms and their efficient implementation in Python, to their application in different fields.
This master class is based on the speaker’s book (co-authored with Omiros Papaspiliopoulos): “An introduction to Sequential Monte Carlo” published by Springer :
https://link.springer.com/book/10.1007/978-3-030-47845-2
The master class was video-recorded and uploaded by CIRM on YouTube:
Workshop on Statistics in Metric Spaces
The workshop on Statistics in Metric Spaces was held at ENSAE, on October 11, 12 and 13, 2023. It brought together international experts in the joint fields of statistics, optimization, probability theory and geometry. Each participant gave a 45-60 min talk and the range of topics that were covered was broad, tackling modern questions concerning statistical analysis on non-standard spaces.
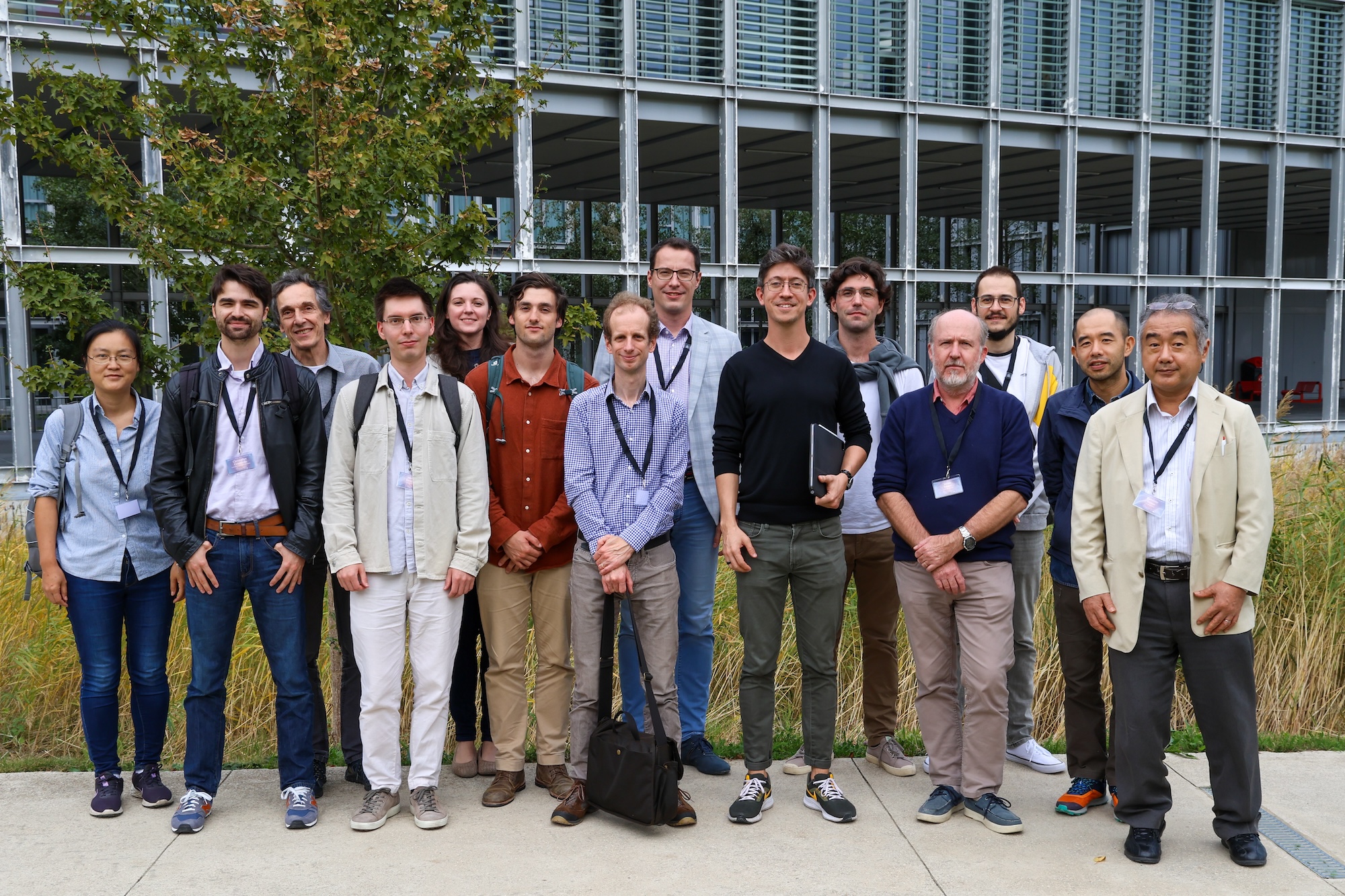
Victor-Emmanuel Brunel (CREST-ENSAE), Christopher Criscitiello (EPFL), Stephan Huckemann (Georg-August-Universität Göttingen), Alexey Kroshnin (Weierstrass-Institut Für Angewandte Analysis und Stochastik), Kazuhiro Kuwae (Fukuoka University), Tom Nye (Newcastle University), Shin-ichi Ohta (Osaka University), Miklós Pálfia (Corvinus University of Budapest), Pierre Pansu (Université Paris-Saclay), Quentin Paris (HSE University), Xavier Pennec (INRIA), Gabriel Romon (CREST-ENSAE), Jordan Serres (CREST-ENSAE), Austin Stromme (CREST-ENSAE)
While available data become more and more rich and complex, it is essential to understand their intrinsic geometry, for instance as a tool of dimensionality reduction or, sometimes, in order to produce interpretable statistical procedures. This, however, also comes at a cost, since these geometries may be non-standard (e.g., non-linear and/or non-smooth geometries), yielding new challenges from the points of view of both statistical and algorithmic analysis.
For instance, directional data lie on spheres or projective spaces. In shape statistics, data are encoded as landmarks on three-dimensional objects, which should be invariant under rigid transformations: Hence, data lie in the quotient of a Euclidean space by a class of rigid transformations. In fact, such quotient spaces are also useful to understand statistical models that arise in econometrics, when a parameter is only identifiable up to some known transformations. Optimal transport theory is based on Wasserstein spaces, which are metric spaces with Riemannian/Finsler-like geometries. In various fields, in particular physics and economics, the geometry provided by optimal transport on sets of probability measures has been shown to be very well adapted to understand general phenomena, such as transportation of goods, or distribution of tasks, capital, etc. In the machine learning community, it has also been recently pointed out that metric trees and hyperbolic spaces, which exhibit negative curvature, are well adapted to encode data with hierarchical structures.
While probability theory is now fairly well understood in smooth, finite dimensional spaces (such as Euclidean spaces and Riemannian manifolds), much less is known in more general metric spaces, exhibiting possible infinite dimension (such as functional spaces), inhomogeneous structure (such as stratified spaces), etc. From a more algorithmic prospective, gradient flows and their discretization in non-smooth spaces are challenging because they require brand new approaches (e.g., new definitions of (sub)-gradients), yet they are essential in order to extend fundamental tools such as gradient descent algorithms to non-standard setups. Even in smooth spaces, the impact of curvature on gradient descent algorithms is still not clearly understood. More generally, the notion of convexity, which is pervasive to probability theory, statistical learning and optimization, and its interplays with curvature, still raise challenging questions.
To summarize, the impact of curvature (or generalized notions of curvature) on measure concentration, on the statistical behavior of learning algorithms and on their computational aspects is a flourishing topic of research that brings together experts in smooth/non-smooth geometry, statistics, probability theory and optimization.
The workshop brought these challenges onto the stage, and yielded fruitful discussions among the participants and the audience, with the goal of entailing future collaborations. We hope that this workshop, on Statistics in Metric Spaces, was the first edition of a long series, that will also spread interest in these rich topics into a broader audience.