27/06/2025
Peter Tankov & David Zerbib for Polytechnique Insights
Deux chercheuses du CREST nommées au Conseil d’analyse économique
Nous sommes heureux d’annoncer la nomination d’Emmanuelle Taugourdeau et de Pauline Rossi, toutes deux chercheuses au CREST, au sein du Conseil d’analyse économique (CAE). Cette reconnaissance illustre l’engagement du laboratoire dans le débat public et la production d’une recherche rigoureuse au service des politiques économiques.
Deux économistes reconnues
Emmanuelle Taugourdeau est directrice de recherche CNRS au CREST et professeure associée à l’École polytechnique. Spécialiste d’économie publique, ses travaux portent sur la fiscalité, les finances publiques locales et la compensation écologique. Elle est également directrice adjointe du CREST et Présidente du comité AFSE WinE (Women In Economics).
Pauline Rossi est professeure à l’École polytechnique et chercheuse au CREST. Elle travaille sur des sujets d’économie du développement, de démographie et d’économie de la famille. Lauréate d’un ERC Starting Grant, elle s’intéresse notamment à la prise de décision en matière de fécondité et aux politiques sociales dans les pays en développement.
Le Conseil d’analyse économique : un pont entre la recherche et la décision publique
Créé en 1997, le Conseil d’analyse économique est un organe placé auprès du Premier ministre. Il a pour mission de produire des analyses indépendantes, fondées sur la recherche scientifique, sur des sujets économiques d’intérêt public. Son rôle est de nourrir la décision publique grâce à des diagnostics rigoureux et accessibles, tout en participant activement aux débats économiques contemporains.
Le CAE publie des notes et rapports sur des thématiques variées : emploi, fiscalité, éducation, climat, inégalités, innovation, etc. Ses travaux sont consultables en ligne et largement relayés dans le débat public.
Être membre du CAE : une responsabilité scientifique et citoyenne
Les membres du CAE sont des économistes de haut niveau, issus du monde académique ou institutionnel. Leur rôle est de proposer des contributions éclairées, en lien avec leurs domaines d’expertise, en collaboration avec les autres membres du conseil. Ces travaux peuvent donner lieu à des recommandations concrètes à destination des pouvoirs publics.
Être nommé·e au CAE, c’est donc :
- Participer à l’élaboration des politiques économiques nationales,
- C à diffuser la recherche en économie dans le débat public,
- Défendre une approche scientifique, rigoureuse et pluraliste de l’analyse économique.
Une reconnaissance forte pour le CREST
Ces nominations sont une fierté pour le CREST, qui voit deux de ses chercheuses rejoindre une instance stratégique au plus haut niveau de l’État. Elles témoignent de la qualité scientifique de nos travaux, de leur pertinence pour les enjeux de société, et de notre contribution active au dialogue entre science et politique.
Les Mouettes Savantes : mathématiques et informatique au service de l’écologie
Du 16 au 20 juin 2025, la deuxième édition des Mouettes Savantes a réuni 30 élèves de seconde issues de lycées de Brest, Rennes et du sud de Paris.
Au programme de ce projet scientifique conçu et organisé par cinq chercheuses et enseignantes-chercheuses, dont Marie Etienne (ENSAI-CREST) : découverte de la recherche en mathématique au service des transitions environnementales, à la station biologique de l’Université de Rennes, en forêt de Paimpont.
Bilan carbone de l’IA générative, alimentation, évolution des températures, moustique tigre et pêche durable : les matinées ont été consacrées à des ateliers scientifiques mobilisant des compétences en mathématiques et informatique, appliquées à des enjeux environnementaux actuels. L’après-midi, place aux activités sportives, ludiques et culturelles au cœur de la forêt de Brocéliande.
Conclusion ? Un franc succès pour ce séjour dont l’objectif est de renforcer la présence des jeunes filles dans les filières et carrières scientifiques.
Marie Etienne a présenté le projet Les Mouettes Savantes lors des Journées Parité de la communauté mathématique 2025 qui se se sont tenues les 23 et 24 juin à l’Université de Rennes, campus Beaulieu.
Ouest France a couvert la dernière édition des Mouettes Savantes dans l’édition du 21 juin 2025.
En savoir plus sur le projet Les Mouettes Savantes
Quelle place pour les dépenses de défense dans les finances publiques ?
Note IPP n°116 – juin 2025
Dans cette note, Pierre Boyer et ses coauteurs proposent une analyse originale des dépenses de défense sous l’angle des finances publiques et de l’économie politique.
La défense est bien un bien public pur : elle bénéficie ainsi à tous, mais toutefois des enjeux d’équité sont soulevés par son financement. D’après les auteurs, les préférences citoyennes varient selon les revenus. En outre, le type d’impôt (TVA, impôt sur le revenu, dette) affecte l’adhésion politique à une augmentation des dépenses.
Ils illustrent la nécessité d’un design fiscal redistributif afin de bâtir un consensus démocratique via des outils de micro-simulation appliqués à la France et à l’Allemagne. À l’échelle européenne, la coordination des budgets pourrait améliorer l’efficacité, à condition de dépasser le risque de « passager clandestin ».
📘 À lire ici : Note complète – IPP n°116
VoxEU Column – Part-time work slows the narrowing of France’s lifetime gender earnings gap – Bertrand Garbinti Cecilia García-Peñalosa Vladimir Pecheu Frederique Savignac
VoxEU Column – Part-time work slows the narrowing of France’s lifetime gender earnings gap – Bertrand Garbinti Cecilia García-Peñalosa Vladimir Pecheu Frederique Savignac – 22 Jun 2025
Arnak Dalalyan awarded an ERC Advanced Grant for his work on generative AI models
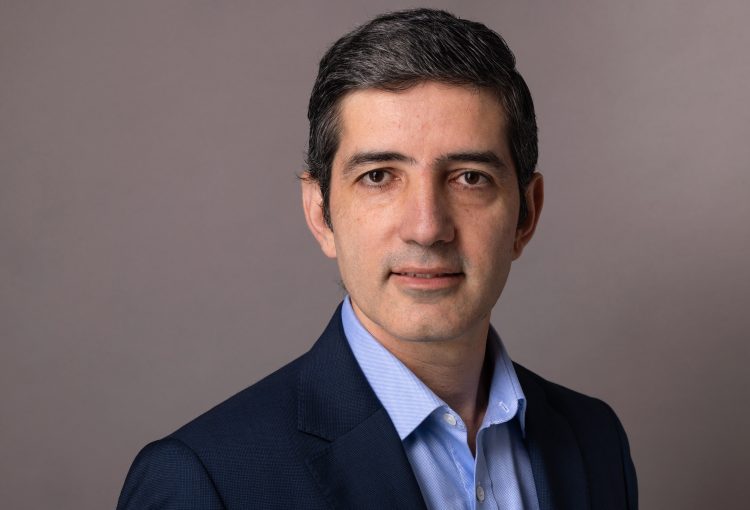
We are proud to announce that Arnak Dalalyan, director of CREST, Hi! PARIS Fellow and Professor of statistics at ENSAE Paris, has been awarded a European Research Council (ERC) Advanced Grant for his project SAGMOS – Statistical Analysis of Generative Models: Sampling Guarantees and Robustness. This highly competitive grant will support his ambitious research at the intersection of statistics, machine learning, and artificial intelligence.
With this new grant, the CREST now hosts eight ongoing ERC projects: three ERC Starting Grants, three ERC Consolidator Grants, and now two ERC Advanced Grants. This remarkable track record reflects the strength, diversity, and international visibility of fundamental and applied research at the lab. Arnak Dalalyan joins a long line of CREST researchers distinguished by the ERC, following for example the recent ERC Consolidator Grant obtained by Yves Le Yaouanq in economics.
About Arnak Dalalyan
A mathematician and statistician, Arnak Dalalyan studied at Yerevan State University (Armenia) before obtaining a PhD in statistics from Le Mans University in 2001, and an HDR from Sorbonne University (formerly Université Pierre et Marie Curie) in 2007. After postdoctoral research at Humboldt University in Berlin and several academic positions in France, he joined ENSAE Paris and CREST, where he has been director since 2020. His research focuses on mathematical statistics and its applications to machine learning, with particular emphasis on robust methods, high-dimensional data, and sampling techniques.
SAGMOS – Statistical Analysis of Generative Models: Sampling Guarantees and Robustness
Generative models — algorithms capable of creating realistic texts, images, music, or molecular structures — are now at the heart of many technological innovations, from artistic creation to drug discovery. The SAGMOS project will provide new mathematical guarantees on the reliability, originality, and efficiency of such models, particularly diffusion models that have become the new standard in the field.
“In recent years, generative models have made remarkable progress. But we still need to understand how much data is required for these models to generate reliable and truly novel outputs. The SAGMOS project aims to bridge this gap by providing precise theoretical guarantees,” explains Arnak Dalalyan.
The project will lead to the recruitment of PhD students and postdoctoral researchers, and to the organisation of international workshops to disseminate its findings.
The ERC Advanced Grant
The ERC Advanced Grant is one of the most prestigious funding schemes in Europe, supporting established researchers with a track record of significant research achievements. The grant allows recipients to pursue ground-breaking, high-risk projects that can lead to major scientific advances. The funding awarded to Arnak Dalalyan reflects the European commitment to frontier research and the scientific excellence of the CREST research centre.
VoxEU Column – Fiscal sustainability and budgetary inertia: When checks and balances really matter – Facundo Piquillem, Alessandro Riboni – 11 Jun 2025
Impôts et perception : l’expertise du CREST mise à contribution
Le Conseil des prélèvements obligatoires (CPO) vient de publier la note n°11 (juin 2025) présentant les analyses complémentaires de la deuxième édition du Baromètre des prélèvements fiscaux et sociaux. Ce travail inédit repose sur un appareil statistique sophistiqué mêlant données déclaratives et données administratives. Il bénéficie de l’expertise de chercheurs du CREST, fortement mobilisés depuis la première édition de 2021.
Une collaboration au service d’une meilleure compréhension du consentement à l’impôt
Le Baromètre du CPO, mené en partenariat avec Harris Interactive, l’Insee et le CREST, vise à mieux comprendre l’opinion des Français sur les impôts et cotisations sociales. Pour la première fois, les réponses des enquêtés ont été appariées à leurs données fiscales réelles (revenus, taux d’imposition), dans un cadre sécurisé et anonyme via le CASD, le Centre d’accès sécurisé aux données.
Des résultats éclairants sur la perception de l’impôt
L’étude confirme que la satisfaction vis-à-vis de l’usage de l’argent public reste le facteur le plus déterminant de l’acceptation des prélèvements. Elle révèle également que près de la moitié des Français connaissent avec précision leur revenu fiscal et leur taux d’imposition, une donnée souvent sous-estimée dans les enquêtes classiques.
Les travaux mettent aussi en évidence que la surestimation de son propre taux d’imposition est corrélée à une moindre acceptation de l’impôt, alors que les réductions d’impôt pour dons aux associations sont associées à une plus grande adhésion au système fiscal. À l’inverse, les crédits d’impôt pour garde d’enfants ou emploi à domicile semblent, de manière paradoxale, associés à un moindre consentement.
Le CREST, au cœur de la recherche socio-fiscale
Un groupe de chercheurs du CREST a été impliqué dans toutes les étapes du projet, depuis la conception de l’enquête jusqu’à l’analyse des données appariées. Leur contribution témoigne de la capacité du laboratoire à combiner outils empiriques de pointe et enjeux de société, notamment autour des questions de justice fiscale, de perception citoyenne et de politiques publiques.
Cette collaboration confirme l’importance de produire des indicateurs fiables pour nourrir le débat public, et montre que les sciences sociales quantitatives ont un rôle essentiel à jouer dans l’évaluation des politiques fiscales et sociales.
Navigating the Future of Money: Highlights from the Second (Back To) The Futures of Money Workshop
On 15-16 May 2025, Blockchain@X Research Center — an academic chair of École Polytechnique hosted at CREST — held the second edition of (Back To) The Futures of Money workshop, at the Maison de l’Amérique Latine in Paris.
This two-day interdisciplinary event brought together scholars, policymakers, and industry experts to discuss the evolution of monetary systems, blockchain innovation, and the future of decentralized finance (DeFi). The program featured academic presentations, industry talks, roundtable discussions, and informal exchanges during the breaks and lunches.
The first day explored the economic and geopolitical dimensions of cryptocurrencies. It featured academic presentations by Bruno Biais (HEC Paris), Linda Schilling (Olin Business School), and Cyril Monnet (University of Bern), followed by a roundtable discussion on the geopolitics of payment systems, moderated by Christian Pfister (Université d’Orléans).
In the afternoon, Laurent Camus (Banque de France) introduced the current state of stablecoin regulation, setting the stage for a lively panel discussion with representatives from Circle, Société Générale, and Angle Protocol. The day concluded with academic insights on privacy-preserving payment mechanisms from Agostino Capponi (Columbia University) and on verifying total value locked (TVL) in DeFi from Pietro Saggese (IMT Lucca).
The second day shifted focus to technological perspectives on blockchain and DeFi. It opened with a forward-looking keynote on technology convergence by Sudhir Pai (Capgemini), followed by a session on trust frameworks for AI and blockchains featuring Gilles Fedak (iExec) and Jason Delabays (Zama). The academic segment continued with presentations from Hanna Halaburda (NYU Stern) and Christoph Schlegel (Flashbots), addressing mechanism design and Sybil-resistance on maximal extractable value (MEV).
In the afternoon, Fahad Saleh (University of Florida) and Andrea Canidio (CoW Protocol) presented their perspectives on the interactions between blockchain layers and Ethereum’s immutability. The day concluded with talks from Louis Bertucci (Institut Louis Bachelier), Evgeny Lyandres (Tel Aviv University), and Julien Prat (IP Paris) on lending protocols, quantitative investment strategies, and systemic risk in DeFi.
We extend our sincere thanks to all speakers, panelists, and participants who contributed to the success of this workshop. (Back To) The Futures of Money II reaffirmed the need for interdisciplinary collaboration in navigating the rapidly evolving financial landscape shaped by blockchain technology. We look forward to continuing the conversation in future editions.
The Blockchain@X Research Center at École Polytechnique aims to combine academic excellence with institutional and scientific prestige in order to foster innovation in blockchain. A pioneer in its field and financially supported by Capgemini, Nomadic Labs and Caisse des Dépôts, it brings together scientists in computer science and economics whose research focuses on blockchains and related technologies. The chair also offers a wide range of courses to students at École Polytechnique who wish to learn about this constantly evolving field, and contributes to the organization of international academic conferences such as Tokenomics.
IPP : Entretien avec Bertrand Garbinti, responsable du pôle fiscalité des ménages
03/06/2025
Bertrand Garbinti , chercheur au CREST-GENES, a pris ses fonctions à l’IPP début janvier 2025, en qualité de responsable du pôle fiscalité des ménages. Près de 6 mois après son arrivée, Bertrand Garbinti revient sur ses motivations et les enjeux associés à la direction de ce pôle « historique » de l’IPP.